How to Generate and Validate Product Hypotheses

Every product owner knows that it takes effort to build something that'll cater to user needs. You'll have to make many tough calls if you wish to grow the company and evolve the product so it delivers more value. But how do you decide what to change in the product, your marketing strategy, or the overall direction to succeed? And how do you make a product that truly resonates with your target audience?
There are many unknowns in business, so many fundamental decisions start from a simple "what if?". But they can't be based on guesses, as you need some proof to fill in the blanks reasonably.
Because there's no universal recipe for successfully building a product, teams collect data, do research, study the dynamics, and generate hypotheses according to the given facts. They then take corresponding actions to find out whether they were right or wrong, make conclusions, and most likely restart the process again.
On this page, we thoroughly inspect product hypotheses. We'll go over what they are, how to create hypothesis statements and validate them, and what goes after this step.
What Is a Hypothesis in Product Management?
A hypothesis in product development and product management is a statement or assumption about the product, planned feature, market, or customer (e.g., their needs, behavior, or expectations) that you can put to the test, evaluate, and base your further decisions on. This may, for instance, regard the upcoming product changes as well as the impact they can result in.
A product hypothesis implies that there is limited knowledge. Hence, the teams need to undergo testing activities to validate their ideas and confirm whether they are true or false.

Hypotheses guide the product development process and may point at important findings to help build a better product that will serve user needs. In essence, teams create hypothesis statements in an attempt to:
- improve the offering;
- boost engagement;
- increase revenue;
- find product-market fit quicker;
- or for other business-related reasons.
It's sort of like an experiment with trial and error, yet, it is data-driven and should be unbiased. This means that teams don't make assumptions out of the blue. Instead, they turn to the collected data, conducted market research, and factual information, which helps avoid completely missing the mark.
The obtained results are then carefully analyzed and may influence decision-making. Such experiments backed by data and analysis are an integral aspect of successful product development and allow startups or businesses to dodge costly startup mistakes.
When do teams create hypothesis statements and validate them? To some extent, hypothesis testing is an ongoing process to work on constantly. It may occur during various product development life cycle stages, from early phases like initiation to late ones like scaling.
In any event, the key here is learning how to generate hypothesis statements and validate them effectively. We'll go over this in more detail later on.
Idea vs. Hypothesis Compared
You might be wondering whether ideas and hypotheses are the same thing. Well, there are a few distinctions.
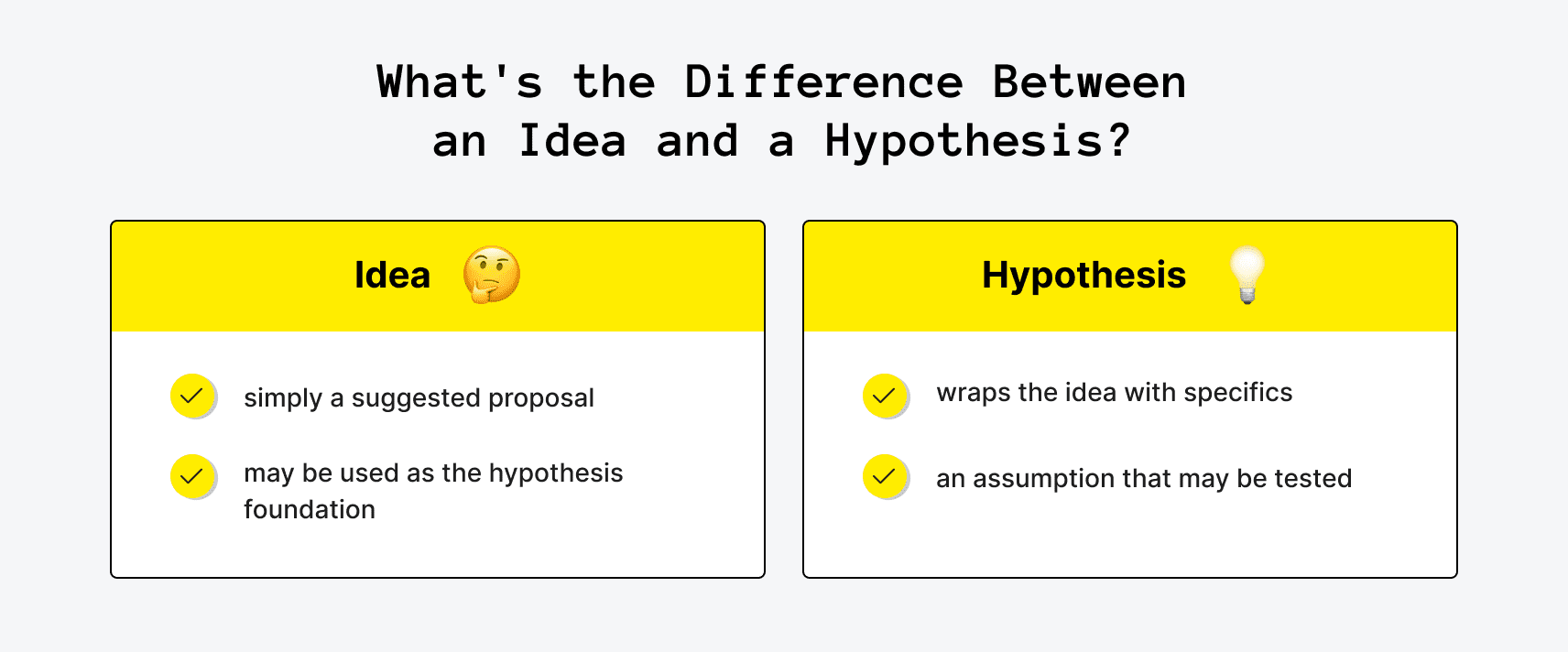
An idea is simply a suggested proposal. Say, a teammate comes up with something you can bring to life during a brainstorming session or pitches in a suggestion like "How about we shorten the checkout process?". You can jot down such ideas and then consider working on them if they'll truly make a difference and improve the product, strategy, or result in other business benefits. Ideas may thus be used as the hypothesis foundation when you decide to prove a concept.
A hypothesis is the next step, when an idea gets wrapped with specifics to become an assumption that may be tested. As such, you can refine the idea by adding details to it. The previously mentioned idea can be worded into a product hypothesis statement like: "The cart abandonment rate is high, and many users flee at checkout. But if we shorten the checkout process by cutting down the number of steps to only two and get rid of four excessive fields, we'll simplify the user journey, boost satisfaction, and may get up to 15% more completed orders".
A product hypothesis is something you can test in an attempt to reach a certain goal. Testing isn't obligatory in this scenario, of course, but the idea may be tested if you weigh the pros and cons and decide that the required effort is worth a try. We'll explain how to create hypothesis statements in the next sections.
3 Fundamental Product Hypothesis Factors
Creating a successful product isn't about guesswork, it's about testing ideas with purpose. And that's what hypothesis driven product development is all about. Instead of relying on hunches, you build clear, testable hypotheses to guide your decisions and improve your product.
But what makes a hypothesis strong? It all boils down to three essential factors that form the backbone of any solid hypothesis.
1. Assumption
Every hypothesis starts with an assumption – your educated guess about what's happening. This could be based on user feedback, analytics, or patterns you've observed. For instance, you might assume users aren't engaging with a specific feature because it's hard to find or doesn't meet their needs. Think of the assumption as the "why" behind your hypothesis.
2. Condition
The condition explains what triggered your assumption. It's the specific context or data that made you notice the problem in the first place. For example, if you see a sudden drop in page traffic or notice users abandoning a particular workflow, that's your condition. It gives your hypothesis grounding in reality and helps prioritize what's worth testing.
3. Prediction (Impact)
Finally, a hypothesis needs a prediction – your expectation of what will happen if your assumption is correct (or incorrect). What will change if you implement a fix or improve a feature? Will user engagement rise? Will page traffic recover? Your prediction sets a clear target for success and ensures you can measure the impact of your efforts.
How to Generate a Hypothesis for a Product
The last thing those developing a product want is to invest time and effort into something that won't bring any visible results, fall short of customer expectations, or won't live up to their needs. Therefore, to increase the chances of achieving a successful outcome and product-led growth, teams may need to revisit their product development approach by optimizing one of the starting points of the process: learning to make reasonable product hypotheses.
If the entire procedure is structured, this may assist you during such stages as the discovery phase and raise the odds of reaching your product goals and setting your business up for success. Yet, what's the entire process like?

Step 1: It all starts with identifying an existing problem. Is there a product area that's experiencing a downfall, a visible trend, or a market gap? Are users often complaining about something in their feedback? Or is there something you're willing to change (say, if you aim to get more profit, increase engagement, optimize a process, expand to a new market, or reach your OKRs and KPIs faster)?
Step 2: Teams then need to work on formulating a hypothesis. They put the statement into concise and short wording that describes what is expected to be achieved. Importantly, it has to be relevant, actionable, backed by data, and without generalizations.
Step 3: Next, they have to test the hypothesis by running experiments to validate it (for instance, via A/B or multivariate testing, prototyping, feedback collection, or other ways).
Step 4: Then, the obtained results of the test must be analyzed. Did one element or page version outperform the other? Depending on what you're testing, you can look into various merits or product performance metrics (such as the click rate, bounce rate, or the number of sign-ups) to assess whether your prediction was correct.
Step 5: Finally, the teams can make conclusions that could lead to data-driven decisions. For example, they can make corresponding changes or roll back a step.
How Else Can You Generate a Product Hypothesis?
Such processes imply digging deep into facts, sharing ideas when a problem is spotted, and studying the possible risks, goals, benefits, and outcomes. You may apply various MVP tools like (FigJam, Notion, or Miro) that were designed to simplify brainstorming sessions, systemize pitched suggestions, and keep everyone organized without losing any ideas.
Additionally, predictive product analysis can also be integrated into this process, leveraging data and insights to anticipate market trends and consumer preferences, thus enhancing decision-making and product development strategies. Another cutting-edge approach involves graph-based RAG, which enhances decision-making by integrating graph data structures for more accurate and contextually relevant results. It fosters a more proactive and informed approach to innovation, ensuring products are not only relevant but also resonate with the target audience, ultimately increasing their chances of success in the market.
Besides, you can settle on one of the many frameworks that facilitate decision-making processes, ideation phases, or feature prioritization. Such frameworks are best applicable if you need to test your assumptions and structure the validation process. These are a few common ones if you're looking toward a systematic approach:
- Business Model Canvas (used to establish the foundation of the business model and helps find answers to vitals like your value proposition, finding the right customer segment, or the ways to make revenue);
- Lean Startup framework (the lean startup framework uses a diagram-like format for capturing major processes and can be handy for testing various hypotheses like how much value a product brings or assumptions on personas, the problem, growth, etc.);
- Design Thinking Process (is all about interactive learning and involves getting an in-depth understanding of the customer needs and pain points, which can be formulated into hypotheses followed by simple prototypes and tests).
Need a hand with product development?
Upsilon's team of pros is ready to share our expertise in building tech products.

How to Make a Hypothesis Statement for a Product
Once you've indicated the addressable problem or opportunity and broken down the issue in focus, you need to work on formulating the hypotheses and associated tasks. By the way, it works the same way if you want to prove that something will be false (a.k.a null hypothesis).
If you're unsure how to write a hypothesis statement, let's explore the essential steps that'll set you on the right track.

Step 1: Allocate the Variable Components
Product hypotheses are generally different for each case, so begin by pinpointing the major variables, i.e., the cause and effect. You'll need to outline what you think is supposed to happen if a change or action gets implemented.
Put simply, the "cause" is what you're planning to change, and the "effect" is what will indicate whether the change is bringing in the expected results. Falling back on the example we brought up earlier, the ineffective checkout process can be the cause, while the increased percentage of completed orders is the metric that'll show the effect.
Make sure to also note such vital points as:
- what the problem and solution are;
- what are the benefits or the expected impact/successful outcome;
- which user group is affected;
- what are the risks;
- what kind of experiments can help test the hypothesis;
- what can measure whether you were right or wrong.
Step 2: Ensure the Connection Is Specific and Logical
Mind that generic connections that lack specifics will get you nowhere. So if you're thinking about how to word a hypothesis statement, make sure that the cause and effect include clear reasons and a logical dependency.
Think about what can be the precise link showing why A affects B. In our checkout example, it could be: fewer steps in the checkout and the removed excessive fields will speed up the process, help avoid confusion, irritate users less, and lead to more completed orders. That's much more explicit than just stating the fact that the checkout needs to be changed to get more completed orders.
Step 3: Decide on the Data You'll Collect
Certainly, multiple things can be used to measure the effect. Therefore, you need to choose the optimal metrics and validation criteria that'll best envision if you're moving in the right direction.
If you need a tip on how to create hypothesis statements that won't result in a waste of time, try to avoid vagueness and be as specific as you can when selecting what can best measure and assess the results of your hypothesis test. The criteria must be measurable and tied to the hypotheses. This can be a realistic percentage or number (say, you expect a 15% increase in completed orders or 2x fewer cart abandonment cases during the checkout phase).
Once again, if you're not realistic, then you might end up misinterpreting the results. Remember that sometimes an increase that's even as little as 2% can make a huge difference, so why make 50% the merit if it's not achievable in the first place?
Step 4: Settle on the Sequence
It's quite common that you'll end up with multiple product hypotheses. Some are more important than others, of course, and some will require more effort and input.
Therefore, just as with the features on your product development roadmap, prioritize your hypotheses according to their impact and importance. Then, group and order them, especially if the results of some hypotheses influence others on your list.
Product Hypothesis Examples
To demonstrate how to create a hypothesis statement and formulate your assumptions clearly, here are several more samples you can use as an example of a hypothesis statement:
- Adding a wishlist feature to the cart with the possibility to send a gift hint to friends via email will increase the likelihood of making a sale and bring in additional sign-ups.
- Placing a limited-time promo code banner stripe on the home page will increase the number of sales in March.
- Moving up the call to action element on the landing page and changing the button text will increase the click-through rate twice.
- By highlighting a new way to use the product, we'll target a niche customer segment (i.e., single parents under 30) and acquire 5% more leads.
How to Validate Hypothesis Statements: The Process Explained
There are multiple options when it comes to validating hypothesis statements. To get appropriate results, you have to come up with the right experiment that'll help you test the hypothesis. You'll need a control group or people who represent your target audience segments or groups to participate (otherwise, your results might not be accurate).
What can serve as the experiment you may run? Experiments may take tons of different forms, and you'll need to choose the one that clicks best with your hypothesis goals (and your available resources, of course). The same goes for how long you'll have to carry out the test (say, a time period of two months or as little as two weeks). Here are several to get you started.

Feedback and User Testing
Talking to users, potential customers, or members of your own online startup community can be another way to test your hypotheses. You may use surveys, questionnaires, or opt for more extensive interviews to validate hypothesis statements and find out what people think. This assumption validation approach involves your existing or potential users and might require some additional time, but can bring you many insights.
Conduct A/B or Multivariate Tests
One of the experiments you may develop involves making more than one version of an element or page to see which option resonates with the users more. As such, you can have a call to action block with different wording or play around with the colors, imagery, visuals, and other things.
To run such split experiments, you can apply tools like VWO that allows to easily construct alternative designs and split what your users see (e.g., one half of the users will see version one, while the other half will see version two). You can track various metrics and apply heatmaps, click maps, and screen recordings to learn more about user response and behavior. Mind, though, that the key to such tests is to get as many users as you can give the tests time. Don't jump to conclusions too soon or if very few people participated in your experiment.
Build Prototypes and Fake Doors
Demos and clickable prototypes can be a great way to save time and money on costly feature or product development. A prototype also allows you to refine the design. However, they can also serve as experiments for validating hypotheses, collecting data, and getting feedback.
For instance, if you have a new feature in mind and want to ensure there is interest, you can utilize such MVP types as fake doors. Make a short demo recording of the feature and place it on your landing page to track interest or test how many people sign up.
Usability Testing
Similarly, you can run experiments to observe how users interact with the feature, page, product, etc. At times, such experiments may be held on prototype testing platforms with a focus group representing your target visitors.
By showing a prototype or early version of the design to users, you can view how people use the solution, where they face problems, or what they don't understand. This may be very helpful if you have hypotheses regarding redesigns and user experience improvements before you move on from prototype to MVP development.
You can even take it a few steps further and build a barebone feature version that people can really interact with, yet you'll be the one behind the curtain to make it happen. There were many MVP examples when companies applied Wizard of Oz or concierge MVPs to validate their hypotheses.
Or you can actually develop some functionality but release it for only a limited number of people to see. This is referred to as a feature flag, which can show really specific results but is effort-intensive.
What Comes After Hypothesis Validation?
Analysis is what you move on to once you've run the experiment. This is the time to review the collected data, metrics, and feedback to validate (or invalidate) the hypothesis.
You have to evaluate the experiment's results to determine whether your product hypotheses were valid or not. For example, if you were testing two versions of an element design, color scheme, or copy, look into which one performed best.
It is crucial to be certain that you have enough data to draw conclusions, though, and that it's accurate and unbiased. Because if you don't, this may be a sign that your experiment needs to be run for some additional time, be altered, or held once again. You won't want to make a solid decision based on uncertain or misleading results, right?

- If the hypothesis was supported, proceed to making corresponding changes (such as implementing a new feature, changing the design, rephrasing your copy, etc.). Remember that your aim was to learn and iterate to improve.
- If your hypothesis was proven false, think of it as a valuable learning experience. The main goal is to learn from the results and be able to adjust your processes accordingly. Dig deep to find out what went wrong and look for patterns and things that may have skewed the results. But if all signs show that you were wrong with your hypothesis, accept this outcome as a fact, and move on. This can help you make conclusions about how to formulate your product hypotheses better next time.
Don't be too judgemental, though, as a failed experiment might only mean that you need to improve the current hypothesis, revise it, or create a new one based on the results of this experiment, and run the process once more to use it as testing knowledge.
On another note, make sure to record your hypotheses and experiment results. Some companies use CRMs to jot down the key findings, while others use something as simple as Google Docs. Either way, this can be your single source of truth that can help you avoid running the same experiments or allow you to compare results over time.
Have doubts about how to bring your product to life?
Upsilon's team of pros can help you build a product most optimally.

Final Thoughts on Product Hypothesis Generation and Validation
The hypothesis-driven approach in product development is a great way to avoid uncalled-for risks and pricey mistakes. You can back up your assumptions with facts, observe your target audience's reactions, and be more certain that this move will deliver value.
However, this only makes sense if the validation of hypothesis statements is backed by relevant data allowing you to determine whether the hypothesis is valid or not. By doing so, you can be certain that you're developing and testing hypotheses to accelerate your product management and avoiding decisions based on guesswork.
Certainly, a failed experiment may bring you just as much knowledge and findings as one that succeeds. Teams have to learn from their mistakes, boost their hypothesis generation, and make improvements according to the results of their experiments. This is an ongoing process, of course, as no product can grow if it isn't iterated and improved.
If you're only planning to or are currently building a product, Upsilon can lend you a helping hand. Our team has years of experience providing product development services for growth-stage startups and building MVPs for early-stage businesses, so you can use our expertise and knowledge to dodge many mistakes. Don't be shy to contact us to discuss your needs!
to top